MI-HEAD - NorHEAD - Norwegian Centre for Headache Research
Machine Intelligence in Headaches – MI-HEAD
MI-HEAD is a large-scale interdisciplinary research project combining clinical headache research, artificial intelligence and machine learning. The overall goal of MI-HEAD is to develop machine learning models to improve diagnostics and treatment of headache disorders.
MI-HEAD is funded by the Research Council of Norway.
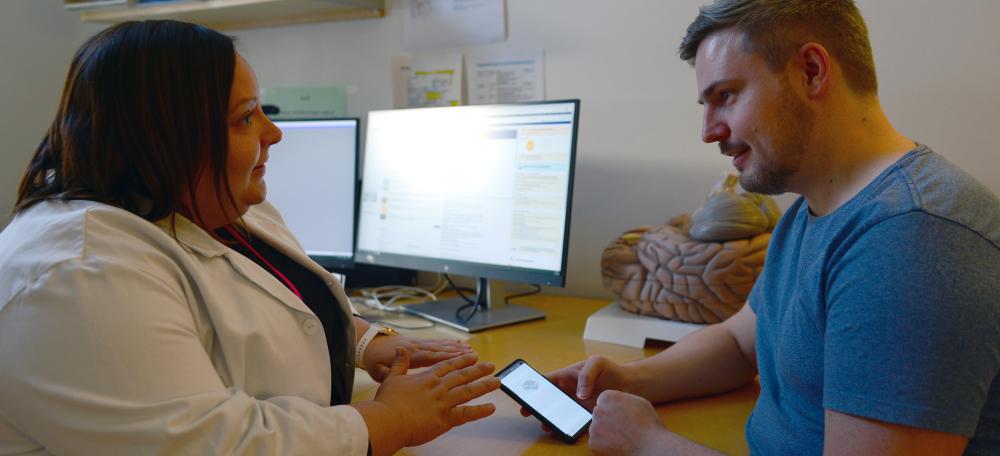
Research
Headache disorders such as migraine, tension-type headache and cluster headache are some of the most burdensome diseases worldwide. Yet, they are often neglected, and patients suffer in pain for a long time before they get a correct diagnosis and effective treatment.
The headache disorders have complex underlying biological mechanisms and symptoms vary considerably from person to person. At present, only a fraction of a person’s symptoms are used for diagnostics, and no simple marker indicates what treatment will be effective at the individual level. This makes it very challenging for the clinician to accurately predict optimal choice of therapy, and repeated trial-and-error is often required to achieve acceptable treatment results.
The goal of the MI-HEAD project is to utilise machine learning to better understand and improve treatment of patients with headaches. Machine learning is a branch of artificial intelligence, where computers are trained to recognize complex patterns in large amounts of data, and make intelligent decisions based on these. Such techniques are especially useful for medical problems where the biological mechanisms are complex to unravel the subtle patterns that explain why some patients respond to certain treatments.
MI-HEAD will combine Norwegian health register data with detailed data from patient’s smartphone headache diaries to create a highly detailed dataset suited for machine learning. By analyzing the large dataset with state-of-the art machine learning models we aim to improve our understanding of why some patients respond to certain types of headache treatments, and others do not.
Moreover, we hope to construct tools that may help the clinician with accurate diagnostics, and effectively predict disease progression and treatment responsiveness. MI-HEAD is organized into four work packages (WP).
Work package 1
In WP1 we will use readily available Norwegian health register data to construct ML models to predict diagnosis, disease progression, and treatment effect in primary headache and draw inferences on underlying neurobiology explaining these predictions.
Work package 2
In WP2 we will implement a novel mobile health tool to prospectively capture large amounts of data that will complement the data collected WP1.
Work package 3
In WP3 we will combine data from WP1 and WP2 to improve the ML models and result in a ML decision support tool that may accurately aid in clinical decision-making in new unseen patients. The goal of the decision support tool is to give qualified recommendations on which
Work package 4
In WP4 we will conduct a randomised controlled trial to evaluate if the developed machine learning tool is superior to standard of care, thus saving patient suffering and socioeconomic expenses.