HYB - Hybrid AI Analytics
Hybrid AI Analytics
- Develop robust, stable and explainable data-driven models for physical systems
- Constrain models to enforce meaningful predictions
- Transfer data-driven models from simulations to reality
- Characterise and quantify uncertainty of data-driven models
This work package will develop methods to predict and reduce the uncertainty of data-driven models. The models will be constrained by existing knowledge, allowing to interpret the model (explainable AI) and reducing the amount of required training data. Applying this methods on real world applications will allow the industry partners to better predict the behavior of their facilities and improve their simulations, e.g. for condition monitoring, predictive maintenance, optimal utilization.
NorwAI has contributed to the theoretical development of a framework for energy-preserving modelling of physical systems through the concept of pseudo-Hamiltonian neural networks. It is based on the theory of port-Hamiltonian descriptions which are often applied in control theory for physical, electrical, or chemical systems.
The code is public on github https://github.com/SINTEF/pseudo-hamiltonian-neural-networks and further described in several papers linked from the repo. The work also forms the basis of demonstrators to be deployed on the Norwegian AI Cloud.
Can competitors cooperate? Yes!
NorwAi partners Kongsberg and Cognite fight in the same market, both companies with unique strengths. Under the guidance of SINTEF Digital the competitors has joined hands to overcome challenges they else would have struggled with. Also other NorwAI partners are looking at the unique cooperation taking place in the work package Hybrid AI Analytics.
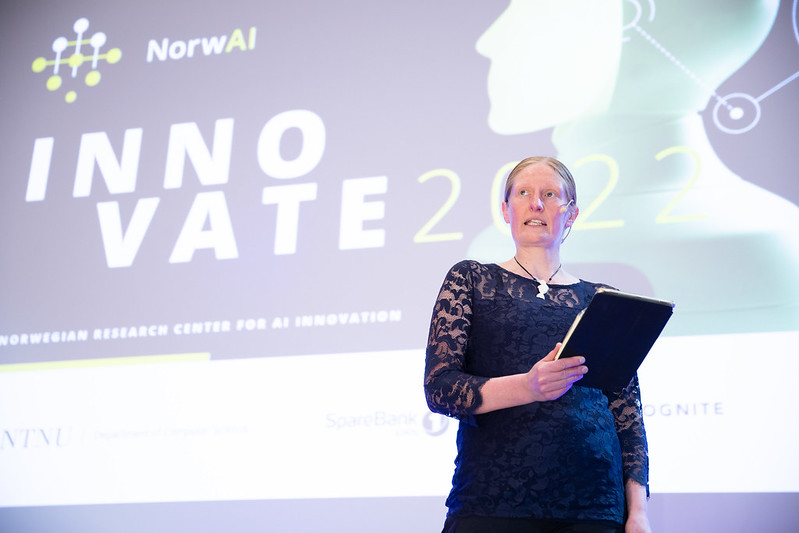
Foto: Kai T. Dragland
2024-02-27
Research stay at Brown university
Katarzyna Michalowska, one of the NorwAI PhD students and researcher at SINTEF Digital is halfway into a one-year research stay at Brown University in the United States as a part of the NorwAI project. Katarzyna shares her experiences so far from working with the CRUNCH group at Brown.

2023-02-09