Exploration efficiency - Bru21
Exploration efficiency
Exploration efficiency
Prototyping future geoscience data organization and analytics tools for improved exploration workflows
Interpreters in exploration assets need a productivity increase in their handling and integrating steadily increasing amounts of data. These terabytes of heterogeneous Big data, including various geophysical data ranging from 2D and 3D surveys with numerous processing versions and large populations of wells, need to be analyzed faster, yet reliably, to provide valuable inputs to decision makers. BRU21 aims at developing novel automated tools to increase data analysis efficiency in the exploration workflows through modern computational methods, e.g. machine learning and artificial intelligence, combined with cross-disciplinary subsurface expertise...read more
Program area team
- Carl Fredrik Berg, Assoc. Prof. Reservoir Engineering
- Karl Wilhelm Johannes Fabian, Professor
- Frank Ove Westad, Adj. Prof. Big Data Cybernetics
- Veronica A.T. Caceres, PhD candidate
- Luc Alberts, PostDoc
- Kurdistan Chawshin, PhD candidate
- Pranav Audhkhasi, PostDoc
- Sushil Acharya, PhD candidate
Projects
Projects
Projects
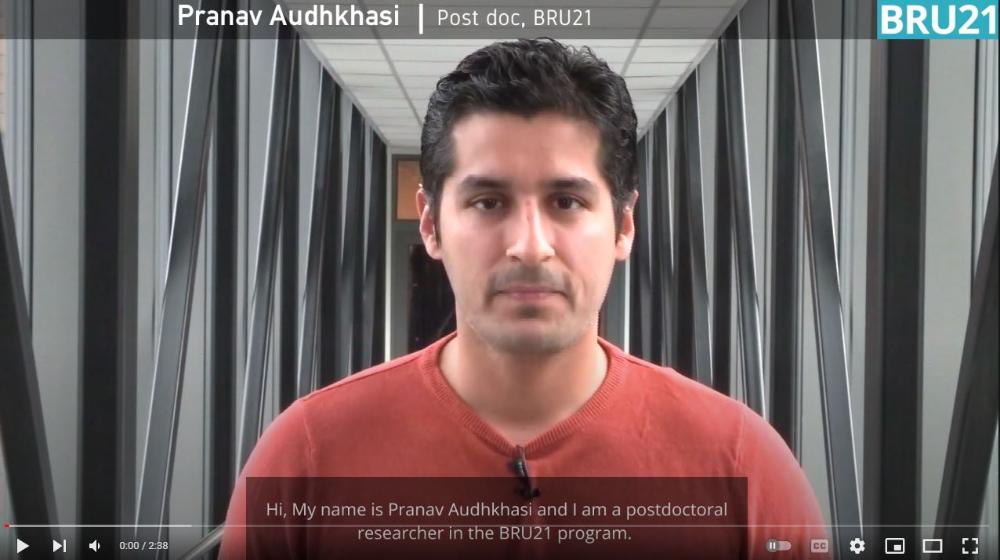
Automated lithofacies classification of pre-stack seismic data

Automated lithology classification employing whole core CT scans
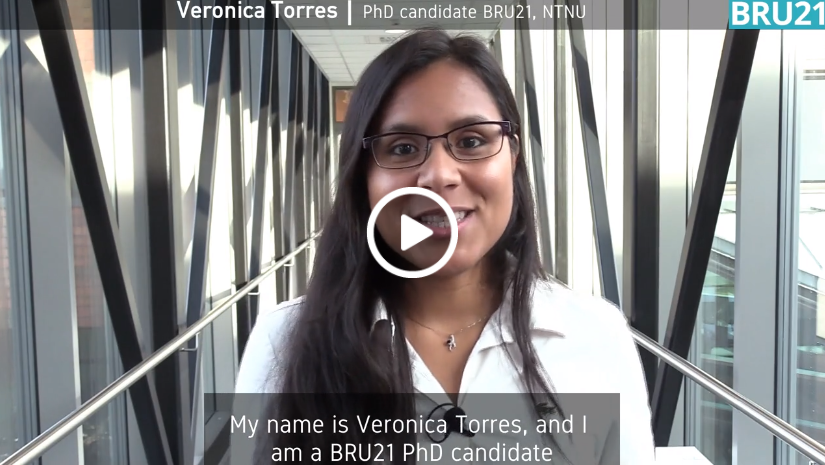
The impact of well data quality on machine learning performance

Automated seismic reconstruction of missing sections
