Reservoir management and production optimization - Bru21
MENU
Reservoir management and production optimization
Reservoir management and production optimization
Development and operation of oil and gas fields require decision-making under uncertainty on several horizons, from field development and optimal reservoir drainage strategies to the day-to-day decisions for operation of the current production infrastructure. BRU21 aims to develop computational tools and strategies to optimize production and manage uncertainty on both longer and shorter horizons. To achieve this, we use learning-based approaches to exploit the abundance of data, and combine with mechanistic models when data lacks information...read more
Program area team
- Lars Imsland, Prof. Optimization and Control
- Carl Fredrik Berg, Assoc. Prof. Reservoir Engineering
- Ashkan Jahanbani Ghahfarokhi, Assoc. Prof. Reservoir Engineering
- Alexey Pavlov, Prof. Petroleum Cybernetics
- Morten Hovd, Prof. Optimizationbased Control
- Damiano Varagnolo, Prof. Statistical Learning and Control
- Jon Kleppe, Prof. Reservoir Engineering
- Tarek Diaa-Eldeen, PhD candidate
- Otávio Fonseca, PhD candidate
- Mammad Mirzayev, PostDoc
- Jinjie Mao, PhD candidate
- Kristian Løvland, PhD candidate
Completed projects:
- Thiago Lima Silva, PostDoc
- Cuthbert Shang Wui Ng, PhD candidate
- Joakim Rostrup Andersen, PhD candidate
- Mathilde Hotvedt, PhD candidate
- Brage Strand Kristoffersen, PhD candidate
Projects
Projects
Projects
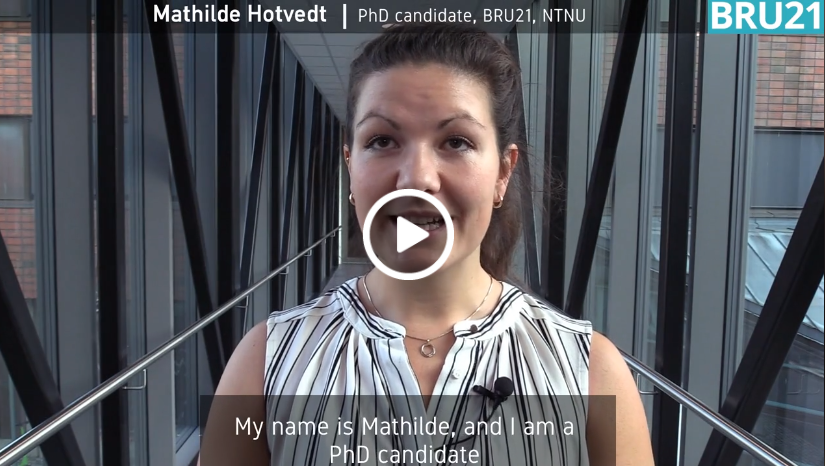
A hybrid data-driven and mechanistic model for production optimization in the oil and gas industry
PhD Candidate Mathilde Hotvedt, Main Supervisor Lars Struen Imsland, Sponsor: Lundin Energy Norway AS
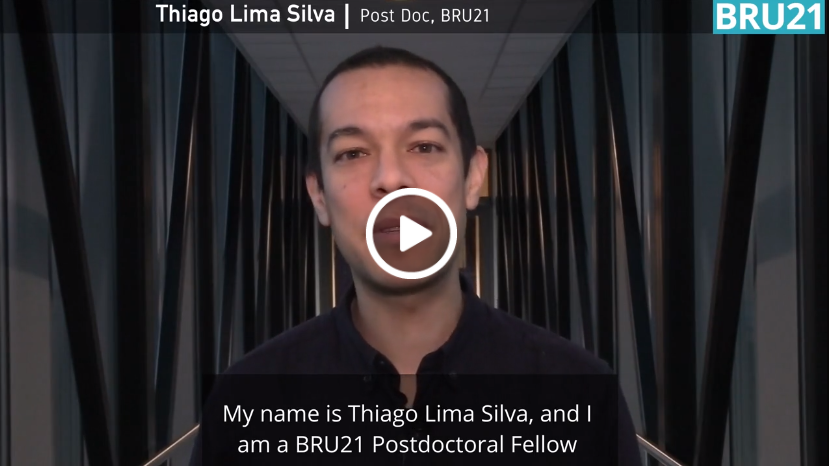
Data-driven control and optimization of oil and gas production systems
Post Doctoral fellow Thiago Lima, Main Supervisor Alexey Pavlov, Sponsor: NTNU
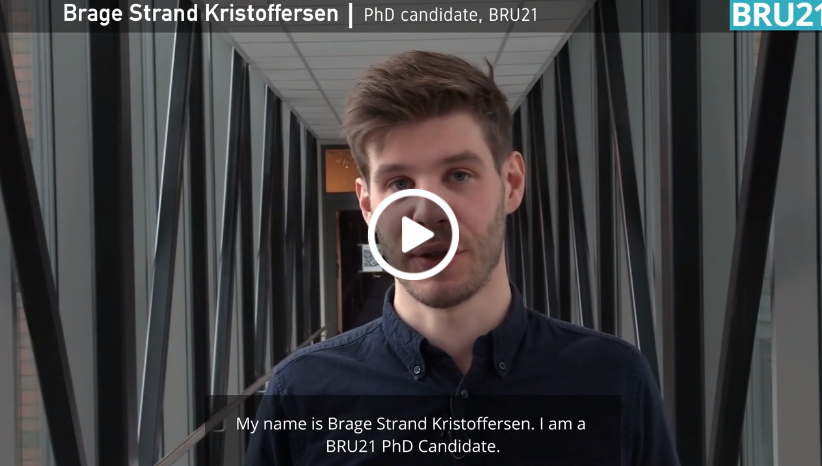
Assisted history matching, reservoir model update and optimization
PhD Candidate Brage Strand Kristoffersen, Main Supervisor Carl Fredrik Berg, Sponsor: Equinor
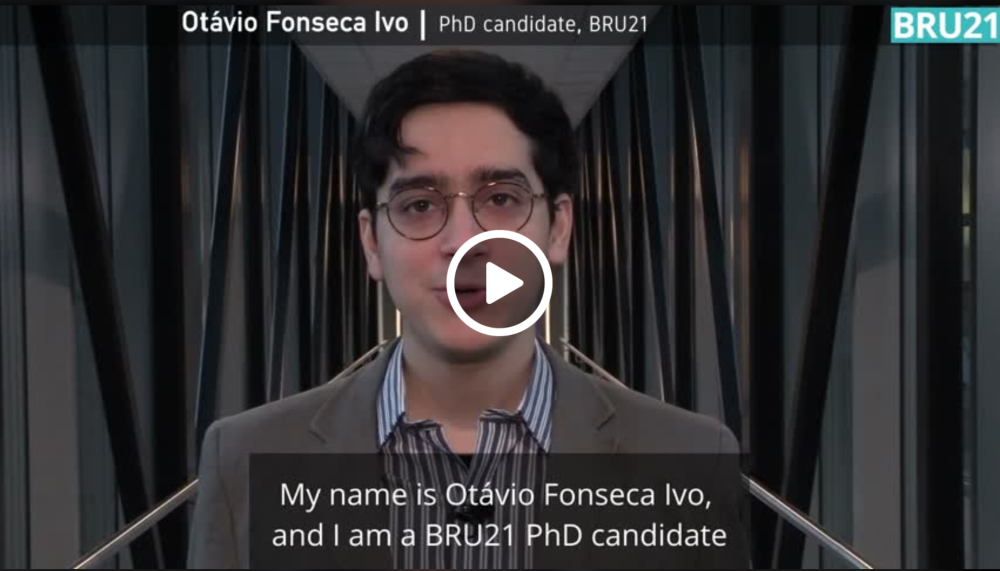
Production optimization strategies for offshore production systems with water processing constraints
PhD Candidate Otavio Ivo, Main Supervisor Lars Struen Imsland, Sponsor: Okea
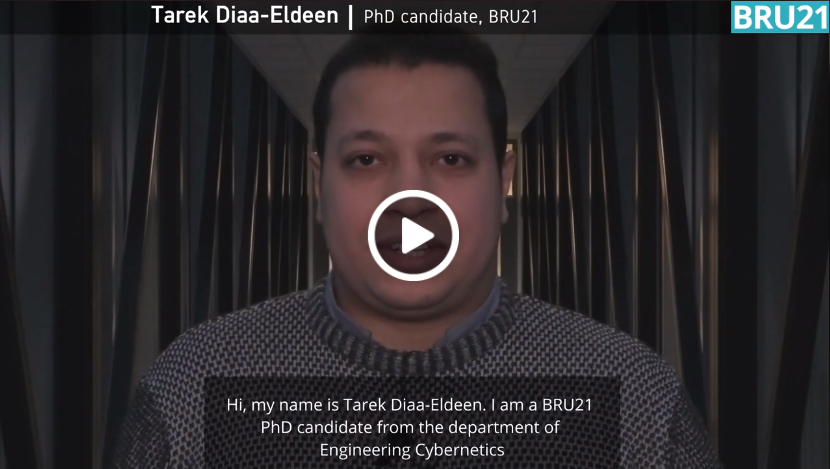
Assisted history matching for petroleum reservoirs
PhD Candidate Tarek Diaa-Eldeen, Main Supervisor Morten Hovd, Sponsor: Equinor
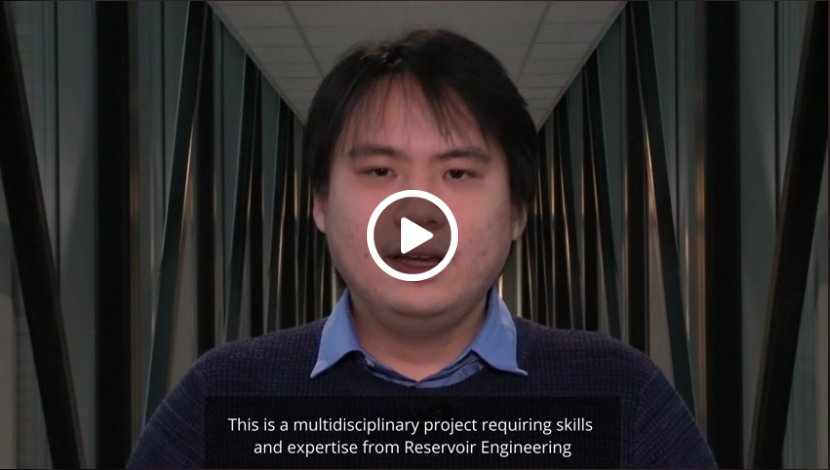
Data-driven reservoir modeling
PhD Candidate Cuthbert Shang Wui NG, Main Supervisor Ashkan Jahanbani Ghahfarokhi, Sponsor: NTNU
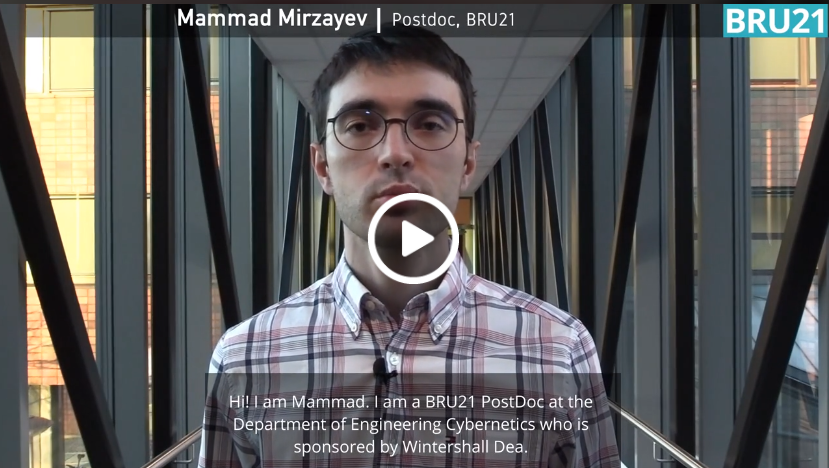
Improved technology for production optimization, with focus on gas lift allocation
Post Doctoral fellow Mammad Mirzayev, Main Supervisor Lars Struen Imsland, Sponsor: Wintershall DEA
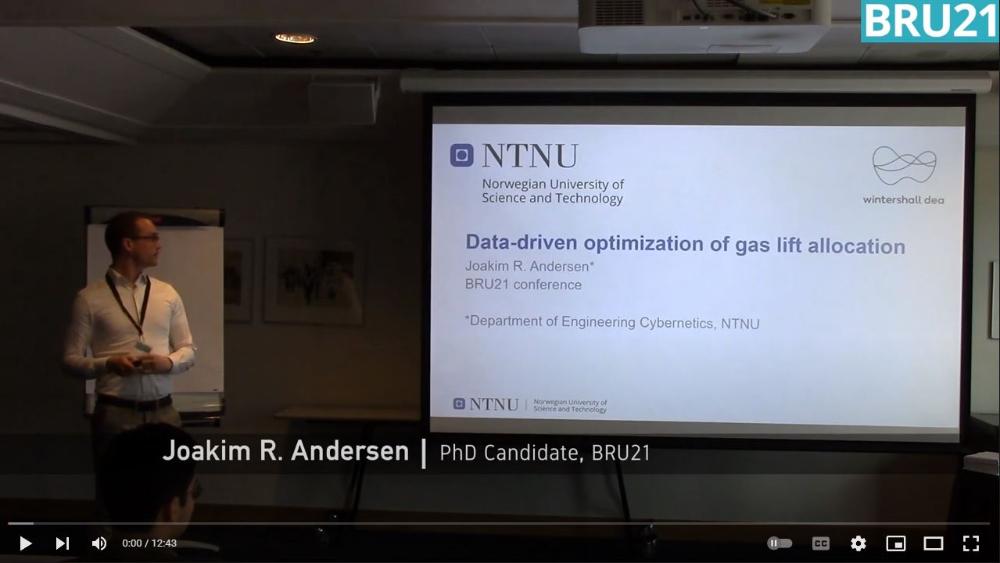
Optimization across time-scales in oil- and gas production
PhD Candidate Joakim Andersen, Main Supervisor Lars Struen Imsland, Sponsor: NTNU
Integrated Reservoir Tool: FieldOpt
Post Doctoral fellow to be hired, Main Supervisor Morten Hovd, Sponsor: NTNU
person-portlet
person-portlet
Program area assistant manager:
Partner access:
Partner access:
-
Projects in Reservoir management and production optimization
-
Reservoir management and production optimization - BRU21 white paper